Harnessing AI-Powered Synthetic Sample to Enhance Diversity in Market Research
October 2, 2024
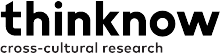
By Mario Carrasco – Co-Founder & Principal
Once seen as an industry resistant to change, market research has embraced transformative technologies in recent years, with AI leading in reshaping traditional methods. Yet, diversity in the data remains elusive, presenting both an opportunity and challenge for researchers. As the founder of ThinkNow, a company at the forefront of multicultural insights, I’ve witnessed firsthand how critical accurate representation is in understanding diverse consumer behavior.
One way we are addressing this disparity is by creating synthetic samples. Over the years, we’ve developed ThinkNow Synthetic—a synthetic sample solution that leverages artificial intelligence to enhance diversity in data collection. However, for synthetic data to advance diversity, the quality of the training data is paramount. This article examines how AI, particularly synthetic sampling, can revolutionize the industry by producing more inclusive and representative datasets, while also highlighting the differences between synthetic sampling and traditional methods like weighting.
The Evolution of Synthetic Sample
Traditional sampling techniques in market research often fall short when it comes to representing hard-to-reach demographics such as Hispanic, Black, AANHPI, and LGBTQIA+ communities. Even with diligent panel recruitment efforts, certain populations remain underrepresented. ThinkNow Synthetic was born out of this necessity, using large language models (LLMs) trained on multicultural data to create synthetic responses that mirror real-world diversity.
The process begins with training the model on diverse datasets, like the General Social Survey (GSS) and ThinkNow’s proprietary data collected from our panel, DigaYGane.com. This ensures that the synthetic sample reflects the population in question and produces responses representing a wide range of cultural experiences. Our approach enhances the inclusiveness of the data and reduces biases often associated with AI-generated responses.
Synthetic Sampling vs. Weighting
A common misconception in market research is to equate synthetic sampling with weighting. While both aim to adjust the data to reflect population diversity better, they employ fundamentally different methodologies. Weighting, as many researchers are familiar with, takes a small sample size and extrapolates the results to a larger population. This can inflate the representation of underrepresented groups but doesn’t truly increase the diversity of responses. Essentially, weighting adjusts the numbers, not the underlying richness or authenticity of the data.
In contrast, synthetic sampling, particularly ThinkNow Synthetic, is designed to create entirely new data points based on the learned behavior of respondents from diverse communities. For example, if you are conducting a study among bicultural Latinos and face difficulty recruiting sufficient respondents, our AI model can generate synthetic responses that mimic those of a bicultural Latino based on actual data collected from our panel. This method doesn’t simply inflate responses but creates new, culturally nuanced data that enriches the overall dataset.
This difference is significant. Weighting amplifies a limited dataset, while synthetic sample expands it by simulating a broader range of responses. This approach has the potential to dramatically increase representation without sacrificing data accuracy.
The Role of Diverse Training Data
The success of any synthetic sample solution hinges on the quality and diversity of its training data. If the training data used to create synthetic responses is skewed or biased, the results will reflect those same biases. Training LLMs on rich, multicultural datasets ensures that synthetic responses are representative and culturally relevant, effectively mitigating the biases often found in AI-generated content.
ThinkNow Synthetic’s hybrid model combines panel data and synthetic responses to create complete and representative datasets. When a client comes to us with a quantitative study needing 1,000 completed responses, for example, we can provide 500 actual survey responses from our diverse panel and supplement the remaining 500 using synthetic data generated by our AI(ThinkNow Synapse – Synt…). This hybrid approach preserves the integrity of the study while reducing costs and accelerating delivery.
Applications and Future Potential
Synthetic sampling is still in its early stages, but the potential applications are vast. From understanding consumer trends to informing policy decisions, synthetic sample can provide a fuller picture of societal behaviors across diverse populations. By filling gaps in datasets with culturally relevant synthetic responses, ThinkNow Synthetic helps clients make more informed decisions that reflect the reality of the communities they serve.
This approach also addresses a major challenge in market research: the underrepresentation of marginalized groups. As brands seek to engage diverse audiences, producing accurate and inclusive data authentically has become a business imperative. Synthetic sampling offers a path forward, equipping researchers with the tools to understand these audiences more deeply.
Conclusion
AI-powered synthetic sample has the potential to revolutionize diversity in market research. However, this is only possible if the training data is as diverse as the populations we aim to represent. At ThinkNow, we are committed to using our years of expertise and rich multicultural datasets to ensure that synthetic sampling doesn’t just mimic diversity but truly reflects it. By combining synthetic data with real-world panel responses, we are creating a new era in market research—one where inclusivity and accuracy go hand in hand.
The future of market research is diverse, and with synthetic sample, we’re ensuring that no voice is left unheard.