Diminishing returns
April 10, 2025
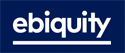
A fundamental concept in marketing effectiveness and media planning
Welcome to The Wheelhouse, where we discuss critical topics for marketers who want to maximise advertising effectiveness.
In this edition, Tom Loughnan, Director of Analytics, explores one of the most fundamental – yet often misunderstood – concepts in media planning: diminishing returns. Tom unpacks why understanding the investment-to-return relationship is essential for making smarter budget decisions, and how the real-world application of econometrics is both powerful and imperfect. From overfitting risks to smart iteration, he offers a clear-eyed view on how to make diminishing returns work in practice.
Understanding the relationship between investment and return is critical in media planning. It seems reasonable to measure this through econometrics, but challenges like overfitting and robustness must be considered. Overfitting becomes more pronounced when factoring in multiple media lines, adstock effects (such as dual transformations), and limited data points.
So, what’s the best way to validate a diminishing returns relationship? The key is iteration.
The law of diminishing returns
The law of diminishing returns is a cornerstone of marketing effectiveness. Everyone familiar with the concept? Just in case you’re not – and to make sure we’re all coming from the same starting point – I’ll define it again: as you invest more in advertising, you generate more sales, revenue, or profit. However, each additional pound spent yields slightly less return than the previous one. This means that the first pound spent is always the most efficient.
Diminishing returns help to explain the efficiency versus scale trade-off, so:
- A low spend results in a modest sales response but a high ROI
- A high spend generates a larger sales response, but efficiency (ROI) declines
What drives diminishing returns?
Several factors contribute to the phenomenon of diminishing returns, including media scalability, audience saturation, and the balance between frequency and reach.
Understanding this relationship is crucial for more effective media planning as it’s the foundation upon which budget recommendations and spend thresholds are set. No matter what happens, once we have the curves, we’re in control.
The role of econometrics in measuring diminishing returns
Econometrics is a powerful, data-driven approach for measuring diminishing returns. For major media channels with significant spend and weekly investment variation, the process is relatively reliable. However, for smaller media lines, measurement precision isn’t always as robust as it appears. Here are four, common measurement challenges.
Limited data at higher spend levels: If a channel has only been used at low weekly spend levels, its impact at medium or high investment levels cannot be reliably measured.
Lack of variation in spend levels: If there are few examples of low or medium spend levels, measurement reliability suffers due to insufficient data points. More data = more reliability.
Ambiguous model outputs: In practice, models often produce either too many statistically significant hypothetical curves or none at all.
Complex interactions between variables:
- We’re not just analysing one media line – we’re measuring all media lines, adstocks, new creative executions, changes in format (e.g. more non-skippable formats), pricing effects, promotional events, competitor activity, weather, and seasonality. This means we’re measuring everything, everywhere, all at once.
- If models are already overfitted – if you have too many variables in your statistical model, as detailed in my colleague Nic Pietersma’s previous post – further inspection of the curve only compounds the problem.
A case in point
Consider the following scenario. Brand X has always used inexpensive, skippable formats on YouTube. For a new campaign launch, we introduce a fresh creative and prioritise non-skippable formats; they cost more and generate more impressions. If our econometric model detects a pattern, what is it truly capturing?
Higher weekly impressions (a genuine effect of diminishing returns)?
The impact of the new creative?
The influence of ad format?
These variables are correlated, making their individual effects difficult to isolate.
The fallacy of precise measurement
Many assume that econometrics, being sophisticated and data-driven, delivers an exact answer. The reality? Marketing Mix Modelling (MMM) provides directional guidance, but the so-called ‘measured’ curves are often heuristics – handy shortcuts, imposed on the model – rather than definitive truths.
Practical approaches to addressing measurement challenges
We recommend the following four approaches to addressing these challenges.
Use sensible assumptions when robust curves cannot be measured:
If your MMM framework is solid, this is likely to be already happening – even if it’s not explicitly stated to stakeholders.
Test and iterate:
Adjust budgets in increments (e.g. increase by 15–20%) and re-evaluate results.
- Collaborate with media agencies and buyers:
- They have deep knowledge of reach potential and buying constraints. Use their insights to inform spend thresholds.
- Leverage benchmarks from similar advertisers:
- Comparing results from similar brands can provide useful directional insights.
- The bigger picture: MMM’s limitations
This challenge extends beyond diminishing returns – it’s a microcosm of the broader issue with MMM. Essentially, we’re using a broad brush to paint in fine detail. While econometrics is an invaluable tool, it must be applied with an understanding of its limitations.
By recognising these constraints and applying a pragmatic approach, we can better navigate the trade-offs between efficiency and scale in media investment.